Case Study January 18, 2022
Optimizing Marketing Spend With Machine Learning
Trexin helped a life sciences company use predictive analytics to improve its marketing campaign performance.
Business Driver
The product marketing team of a large global medical device company had no consistent process for determining which of their numerous customer clinics should receive targeted promotions regarding cardiac patient monitor device upgrades, resulting in inefficient marketing campaigns and lower return on marketing investment. What was known with certainty, however, was that some clinics seemed to respond more quickly and thoroughly than others to device upgrade requests. Recognizing the need to develop a more repeatable, systemic approach to marketing investments, the senior director of marketing asked Trexin to develop a statistical model to reveal the key indicators of clinics with higher device update adoption rates with the goal of providing a foundation for more productive, consistent target marketing decisions.
Approach
Wanting more than just an operational study, our Client asked that the project be structured as a two-month timebox and adopt a minimal viable product approach to concentrate the effort on quickly developing a usable, operational solution. Collaborating directly with the product marketing team and their analytics support staff, Trexin’s data scientists conducted a brief analysis of the data and confirmed there was ambiguity as to why some clinics were more aggressive adopters of upgrade requests than others. Based on that context, Trexin recommended the use of machine learning to drive insights from the data not readily evident with traditional analysis techniques.
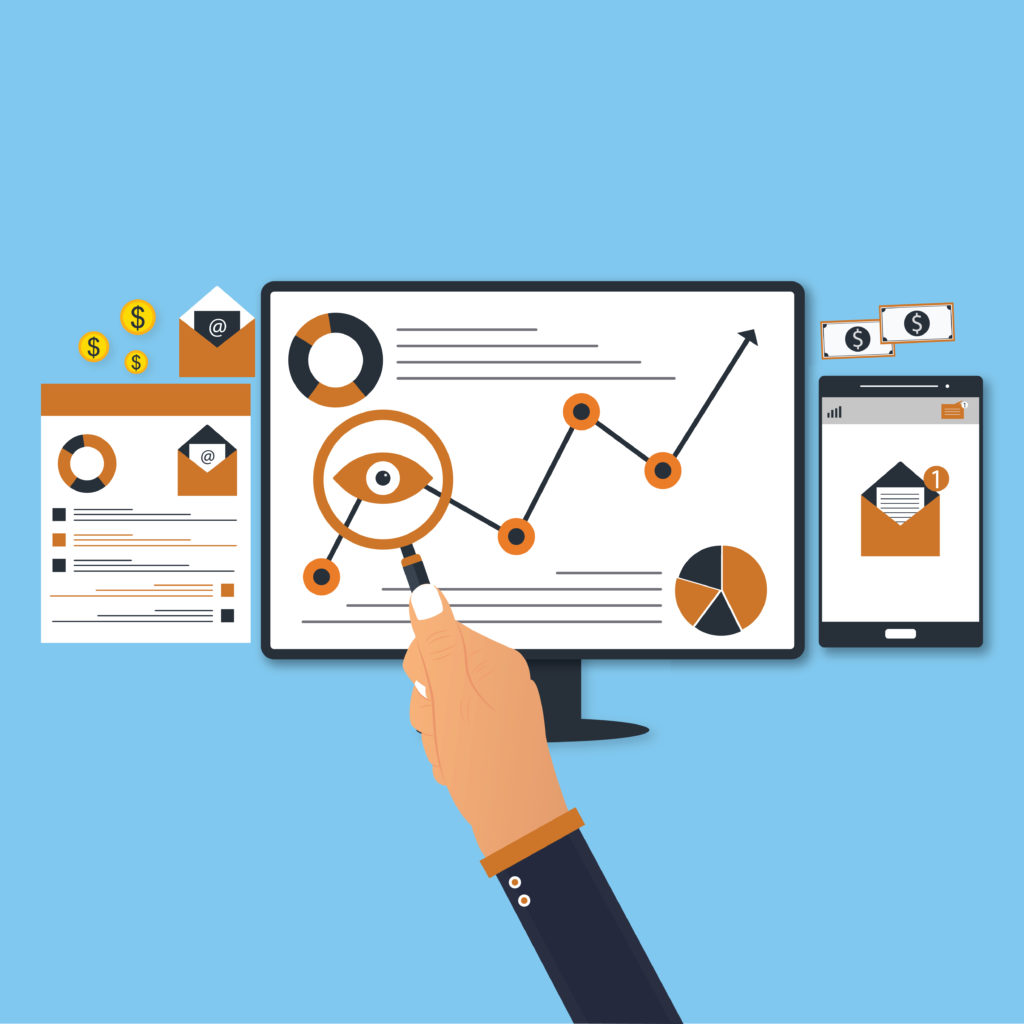
Results
Trexin designed and implemented a machine learning regression-based lasso cross-validation (LCV) model in Python to identify historical key indicators of clinics with higher adoption rates. Considering more than 100 distinct variables that included clinic attributes, device attributes, and demographical attributes (such as rural vs urban locations), the LCV model identified 9 specific key attributes of clinics that had higher historical application adoption rates. Multiple validation steps were used to confirm the results, creating a framework that immediately allowed marketing management personnel to create targeted campaigns during a subsequent company-wide digital transformation initiative for remote cardiac patient monitoring capabilities. The machine learning model was created so that it could apply a uniform methodology for other feature selection and was integrated with data processing and predictive analytics components to create a broader facility that predicts a clinic’s future application adoption rate.